Customer Sentiment Analysis: Definition, Tools, Benefits- 2025
Customer Sentiment Analysis Definition and Meaning
Customer sentiment analysis is a way for businesses to figure out how their customers feel about their products, customer services, or brand. It uses technology—often artificial intelligence (AI)—to scan things like customer reviews, social media posts, survey responses, or emails to determine if people feel positive, negative, or neutral about something.
How It Works:
- Collect Data – Gather feedback from sources like tweets, reviews, or support tickets.
- Analyze Sentiment – Use AI or software to detect emotions and opinions in the text.
- Classify Sentiment – The software sorts feedback into categories:
- Positive (e.g., "I love this product!")
- Negative (e.g., "Terrible customer service.")
- Neutral (e.g., "It's okay, nothing special.")
- Take Action – Businesses use this insight to improve their products, respond to unhappy customers, or promote what people love.
Why It’s Useful:
- Helps companies understand what customers like or dislike.
- Can identify potential issues before they become major problems.
- Allows businesses to respond quickly to customer concerns.
- Improves marketing, product development, and customer service based on real feedback.
In short, sentiment analysis is like a digital "mood detector" for customer opinions, helping businesses make smarter decisions!
Tools to Implement Sentiment Analysis
Popular tools like Crescendo AI, MonkeyLearn, Lexalytics, and IBM Watson streamline sentiment analysis. To get started, organize your feedback data, choose the right tool, and act on insights to improve operations
1. Crescendo AI
Speciality: Customer Support Sentiment Analysis Tool, analyzing support tickets via chat, email, and voice calls.
How it works
Crescendo.ai offers a comprehensive customer sentiment analysis service by integrating advanced artificial intelligence (AI) with human expertise. Here's how they provide this service.
AI-Powered Sentiment Detection: Crescendo's platform utilizes advanced natural language processing (NLP) and machine learning algorithms to analyze customer interactions across various channels—such as chat, email, phone, and messaging. By examining the tone, word choice, and context, the AI detects emotional cues like frustration, satisfaction, or confusion in real-time.
Automated Customer Satisfaction (CSAT) Scoring: Traditionally, CSAT scores rely on customer-completed surveys, which often have low response rates. Crescendo's AI addresses this by automatically assigning CSAT scores to 100% of customer interactions. It evaluates factors such as tone, resolution time, and keyword usage to determine satisfaction levels, providing a more comprehensive understanding of customer sentiment.
Along with providing customer sentiment analysis service, Crescendo AI offers all-in-one customer support services, including
- 10+ advanced analytical tools,
- AI chatbots,
- Email support,
- AI voice assistants, and
- A team of 3,000+ human agents and CX experts that you can use as your extended team at no additional cost.
Crescendo AI combines advanced AI with human expertise for omnichannel support.
Sentimental analysis example:
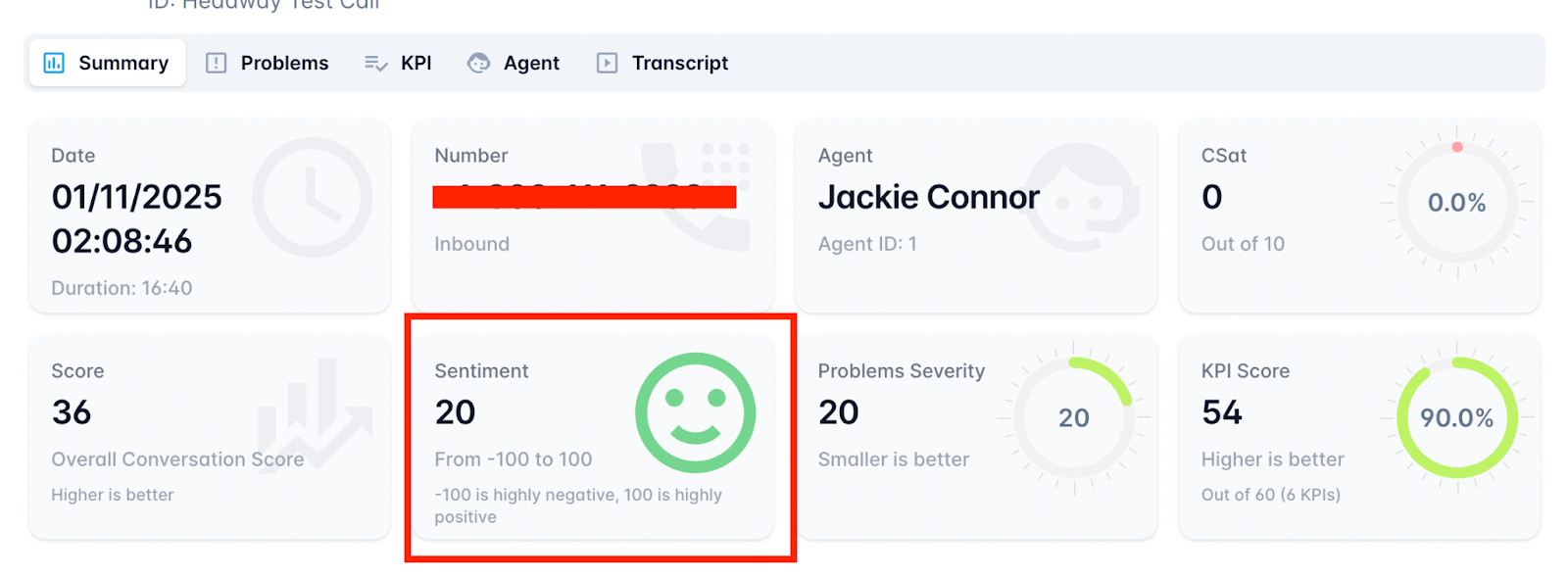
In the above screenshot, Crescendo's AI has calculated the sentimental analysis of a customer support ticket.

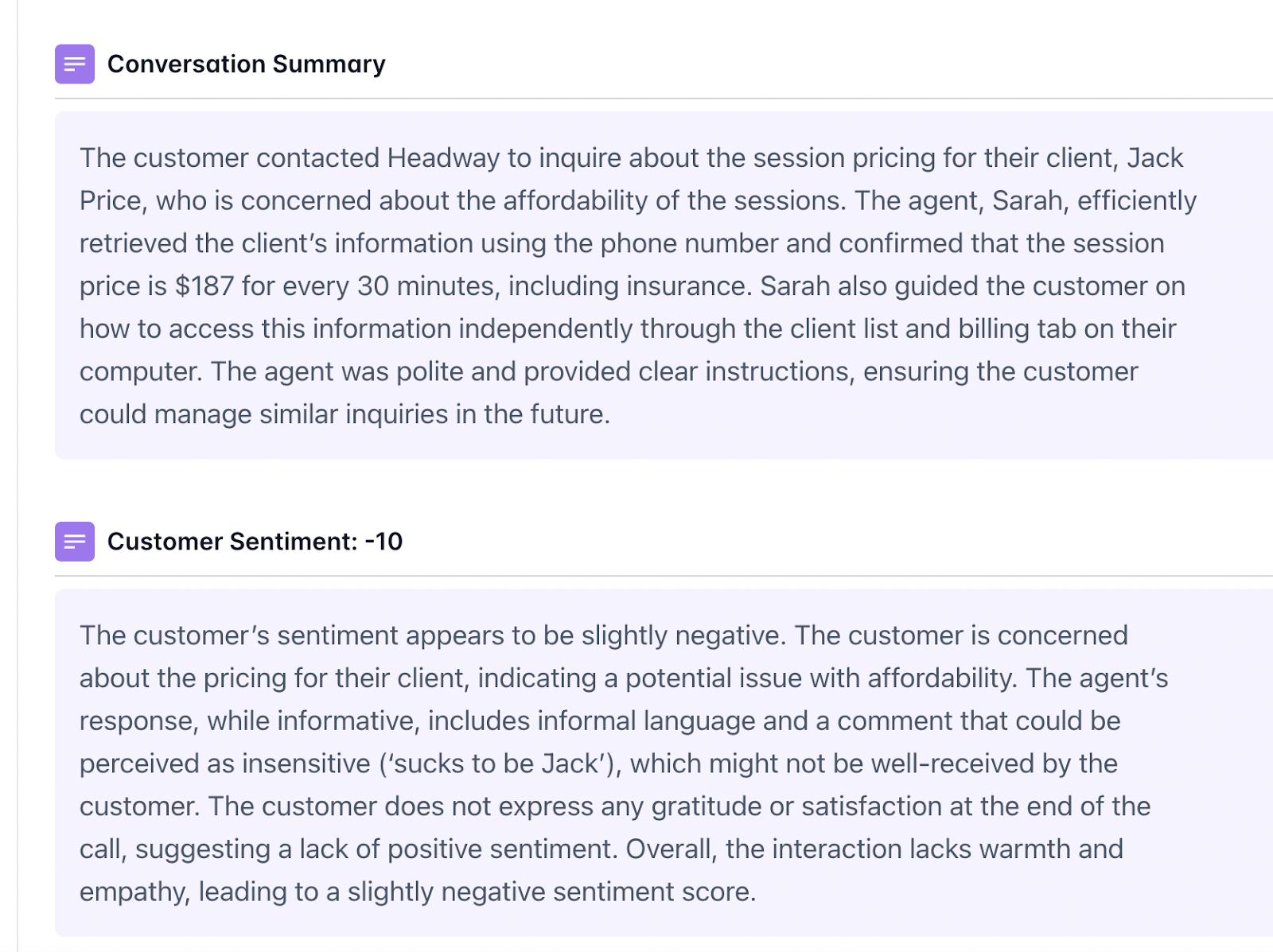
In the above example, Crescendo's AI tools have analyzed a customer support conversation, interpreted what went wrong, and provided recommendations to improve the sentimental analysis score.
2. Meltwater
Meltwater offers a comprehensive sentiment analysis service that enables businesses to understand public perception by analyzing vast amounts of online content.
This analysis is applied to a wide range of sources, including social media platforms, news articles, blogs, and forums. The platform supports sentiment analysis in 218 languages and dialects, allowing businesses to monitor global conversations and understand sentiment across diverse markets.
Multilingual Social Analysis: Meltwater's social listening tools provide real-time monitoring of brand mentions and associated sentiments. Users can set up automated alerts to notify them of sudden changes in sentiment, enabling swift responses to emerging issues or crises.
Visual Sentiment Analysis: Beyond text, Meltwater offers visual enrichments that analyze non-textual content, such as images. This includes recognizing logos, celebrities, emotions, and scenes within images, providing a more holistic view of brand perception.
3. Brand24
Brand24 is a social media monitoring tool that employs advanced artificial intelligence (AI) and machine learning techniques to conduct sentiment analysis, helping businesses understand public perception of their brand, products, or services.
Data Collection: Brand24 continuously monitors various online platforms—including social media sites, blogs, forums, news outlets, and more—to gather mentions of specified keywords related to a brand or topic.
AI-Powered Sentiment Analysis: Utilizing state-of-the-art deep learning and pretrained language models (PLM), Brand24's algorithm analyzes the collected text to determine the sentiment expressed. This involves assessing the emotional tone of the content to classify it as positive, negative, or neutral.
User Interaction and Manual Adjustments: While the AI strives for high accuracy, users can manually adjust the sentiment classification of specific mentions if discrepancies are found, ensuring the data reflects true public perception.
.webp)
In the above screenshot, Brand24's AI tool has analyzed the number of positive and negative comments on a social media site.
4. IBM Watson
IBM Watson employs advanced natural language processing (NLP) and machine learning techniques to perform sentiment analysis through its Watson Natural Language Understanding (NLU) service. Here's how it works.
Data Collection: Watson NLU processes unstructured text data from various sources, such as customer reviews, social media posts, emails, and more. Users input this text into the Watson NLU service for analysis.
Sentiment Analysis: The service analyzes the overall sentiment of the text, determining whether it is positive, negative, or neutral. This helps businesses understand the general tone of customer feedback or public opinion.
Emotion Detection: Beyond basic sentiment, Watson NLU can detect specific emotions such as joy, anger, disgust, sadness, and fear. This provides a deeper understanding of the emotional nuances in the text.
Targeted Sentiment Analysis: Watson NLU offers targeted sentiment analysis, which assesses sentiment toward specific entities or aspects within the text. For example, in the sentence "I love the camera on this phone, but the battery life is disappointing," the service can identify positive sentiment toward the camera and negative sentiment toward the battery life.
Benefits of Customer Sentiment Analysis
Using sentiment analysis can improve customer interactions while influencing product improvements, marketing strategies, and risk management efforts.
1. Better Customer Service
Sentiment analysis helps streamline and improve customer service. In fact, 83% of U.S. consumers say good customer service is critical for brand loyalty [4].
For example,
- James Villas used SentiSum to prioritize urgent cases, reducing resolution times by 51% [1].
- Glammmup saw their Customer Satisfaction Score (CSAT) rise from 68 to 82 within a year by leveraging sentiment analysis [1].
- Lakrids by Bülow identified an increase in packaging complaints through sentiment analysis, updated their packaging, and reduced complaints by 26% by 2021 [1].
"Through using Sentisum we've significantly reduced the time it takes to unearth customer insights. We now understand these at a much more granular level, which allows us to quickly put actions in place to drive improvements." - Joe Quinlivan, Head of Customer Care @ Gousto [1]
2. Product Development Insights
Sentiment analysis also plays a role in refining products. One electronics brand reviewed feedback on its wireless earbuds, uncovering common complaints about fit and battery life. After making improvements, their product ratings jumped from 3.8 to 4.6 [3].
The financial upside of addressing customer feedback is clear:
3. Marketing and Sales Impact
Sentiment analysis can enhance marketing and sales efforts.
- The Atlanta Hawks used Sprout Social to refine their social media approach, leading to a 127.1% increase in video views and a 170.1% growth in their audience within three months [4].
- Expedia Canada reworked a violin-themed TV ad after identifying viewer irritation through sentiment analysis. The revised, humor-driven campaign was a success [5].
- Webconnex discovered that customers were feeling reluctant to make a purchase in the absence of 24/7 multilingual support. After addressing this challenge by partnering with Crescendo, an agentic-AI customer support solution, they achieved over 12,000 additional ticket sales year-over-year.
4. Risk Management
Tracking sentiment can also help identify risks and protect brand reputation.
For instance,
James Hardie used Sprout Social to detect negative sentiment, which informed adjustments to their product development and sales strategies [4].
Customer Sentiment Analysis AI Explained
Setup and Usage Guide
To make the most of sentiment analysis, set up your data correctly, pick the right tools, and use the insights effectively.
Data Setup
Start by organizing your customer feedback for better accuracy. Collect data from sources like social media, customer reviews, support chats, and surveys [10]. Clean it up by removing emojis, punctuation, and unnecessary words.
Tool Selection
Pick sentiment analysis software that fits your business needs. Keep these factors in mind when choosing:
After choosing your tool, use the insights to enhance your operations.
Using Analysis Results
Turn sentiment data into real business changes. For example, a South African bank worked with Repustate to analyze 2 million social media posts in 90 days. They discovered peak complaint times occurred during lunch hours. By adjusting teller staffing, they reduced customer attrition and even attracted new clients [11].
Performance Tracking
To ensure your sentiment analysis is effective, monitor these key metrics:
- Accuracy Assessment: Compare AI-generated classifications with human-verified samples to track false positives and negatives.
- Response Time Monitoring: Measure how quickly your system detects and categorizes changes in sentiment.
- Impact Measurement: Record measurable improvements like higher customer satisfaction scores, faster issue resolution, and cost savings per issue.
Regularly review and adjust your approach to keep improving your results.
Future Development and Ethics
NLP Progress
Natural Language Processing (NLP) continues to evolve, improving both accuracy and context recognition. By 2030, the global NLP market is expected to hit $156.80 billion [14], fueled by advancements in Large Language Models (LLMs) and deep learning.
Here’s a snapshot of key developments:
These innovations are paving the way for deeper integration with other AI technologies, making NLP a cornerstone of modern AI systems.
Ethics and Guidelines
As AI grows more powerful, ethical considerations take center stage. Here are some critical areas to focus on:
One example of ethical AI in action comes from Private AI. While analyzing Starbucks customer reviews, they developed a system to redact employee names while preserving location data. This approach ensured privacy without compromising the accuracy of their insights.
To responsibly harness AI, organizations should:
- Use strong data encryption methods
- Regularly test for and address bias in AI models
- Maintain transparency in decision-making processes
- Train staff on ethical AI practices
- Establish clear governance and accountability frameworks
The future of sentiment analysis depends on balancing progress with ethical responsibility, ensuring that as these tools grow more sophisticated, they remain fair and protect user privacy.
Summary
Understanding customer sentiment is crucial to keeping up with rising customer expectations. For example, 73% of customers want businesses to acknowledge their specific needs [15], and 82% expect responses in under 10 minutes [17]. Clearly, addressing customer emotions is critical.
The business benefits of sentiment analysis are clear:
Real-world examples show how customer sentiment analysis delivers results. T-Mobile uses Natural Language Understanding (NLU) models to process thousands of customer requests daily, solving issues before they escalate [19]. Ford leverages sentiment analysis to gather feedback on vehicle performance, enabling quick product improvements based on real-time insights [19].
These outcomes highlight the importance of incorporating sentiment analysis into customer service. Here are some actionable steps:
- Monitor social media sentiment to engage with a potential audience of 4.76 billion users [17].
- Use sentiment data to enhance customer service training [18].
- Automate feedback collection with surveys [18].
- Align sentiment metrics with key business performance indicators [16].
"Sentiment analysis is an integral part of delivering an exceptional AI customer experience. It helps you understand the nuances of emotion that drive satisfaction, loyalty and advocacy." – Sprout Social [15]