AI Bias: 10 Real AI Bias Examples & Mitigation Guide
What Exactly is AI Bias?
AI bias refers to systematic and unfair discrimination in the outputs of an artificial intelligence system due to biased data, algorithms, or assumptions.
In simple terms, if an AI is trained on data that reflects human or societal prejudices (like racism, sexism, etc.), it can learn and reproduce those same biases in its decisions or predictions.
“Bias is a human problem. When we talk about ‘bias in AI,’ we must remember that computers learn from us.” - Michael Choma.
10 AI Bias Examples in Real Life
Yes, AI bias is very real—and it's not just a small group of individuals who experience its effects; even large corporations have encountered bias in their AI systems and have made significant efforts to identify and eliminate it. Here are some examples of AI bias cases that disrupted the AI industry.
Example 1: Amazon’s AI-Biased Recruitment
Amazon had to scrap an AI-biased recruiting tool after discovering it was downgrading resumes that included the word “women’s,” like “women’s chess club.” It also penalized graduates of all-women's colleges.
It performed worse for female candidates because it was trained on historical hiring data that favored men.
Example 2: AI Bias in the U.S. Justice System
The COMPAS algorithm, developed by Northpointe (now Equivant), is used to predict recidivism risk in U.S. courts. A 2016 ProPublica analysis found that Black defendants were almost twice as likely to be incorrectly classified as high-risk (45%) compared to white defendants (23%).
Conversely, white defendants were more likely to be mislabeled as low-risk, despite reoffending.
These findings exposed significant racial bias in the algorithm, raising concerns about the fairness and transparency of AI tools used in the criminal justice system.
Example 3: Racial AI Bias in the U.S. Healthcare
The study, published in Science, revealed that bias reduced the number of Black patients identified for care by more than 50%.
They discovered that a widely used healthcare algorithm, affecting over 200 million patients in U.S. hospitals, significantly favored white patients over Black patients when predicting who needed extra medical care.
The AI algorithm used healthcare spending as a proxy for need, but because Black patients historically had less access to care and spent less, they were wrongly flagged as lower risk. This led to Black patients receiving less support, despite having equal or greater health needs.
Example 4: Apple’s Gender-Biased AI for Credit Cards
Apple’s credit card algorithm (managed by Goldman Sachs) faced scrutiny after it reportedly offered significantly lower credit limits to women compared to their male spouses, even when women had higher credit scores and incomes.
- Tech entrepreneur David Heinemeier Hansson highlighted that he received a credit limit 20 times higher than his wife's, despite her higher credit score and shared financial accounts.
- Similarly, Apple co-founder Steve Wozniak reported receiving a credit limit ten times greater than his wife's, even though they had joint assets and accounts.
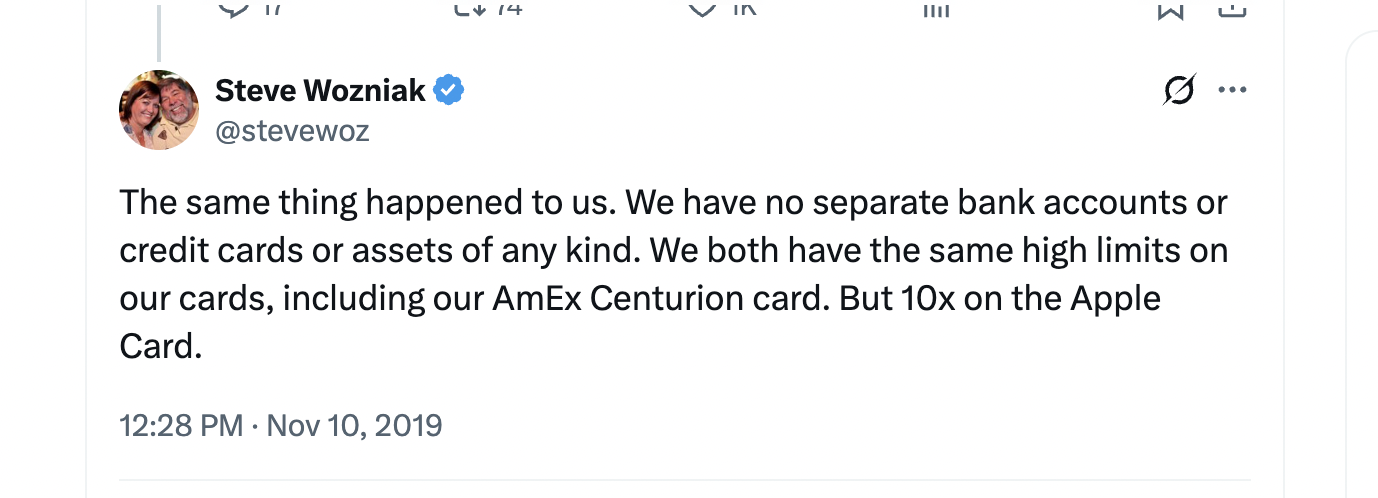
Example 5: AI Bias in Generative AI
Popular generative AI image tools like DALL·E 2 and Stable Diffusion showed significant biases. When asked to generate images for professions such as "CEO" or "engineer," the AI overwhelmingly produced images of white males. Conversely, prompts like "housekeeper" or "nurse" primarily generated images of women or minorities, reflecting stereotypical biases embedded in training data.
In late 2022, the viral Lensa AI app generated stylized avatars based on users' selfies. Users reported gender biases, with female avatars often sexualized or depicted in suggestive poses, regardless of the original image provided. Male avatars, meanwhile, were more frequently portrayed as heroic or powerful, highlighting stereotypical portrayals of gender
Example 6: LinkedIn’s AI-Bias Algorithm in Job Searches
LinkedIn's AI-driven job recommendation systems faced allegations of perpetuating gender biases. A 2022 study introduced a fairness metric to detect algorithmic bias, revealing that LinkedIn's algorithms favored male candidates over equally qualified female counterparts, leading to unequal job recommendations.
Additionally, a 2016 investigation uncovered that LinkedIn's search algorithm suggested male alternatives when users searched for common female names, such as prompting "Stephen Williams" when searching for "Stephanie Williams." These instances highlight the challenges in ensuring AI systems do not reinforce existing societal biases.
The LinkedIn team built a new AI algorithm to deal with this issue.
Example 7: Facebook’s Age-Related AI Bias
ProPublica revealed significant age bias in Facebook's targeted job advertising. Employers were able to exclude older workers from viewing job listings by restricting ad visibility to younger age groups, primarily individuals under 40.
This targeting prevented older adults from accessing opportunities, violating anti-discrimination laws like the Age Discrimination in Employment Act (ADEA).
Companies such as Verizon and Amazon faced legal scrutiny for using this feature.
Facebook subsequently changed its ad policies and settled multiple lawsuits, committing to prevent age-based discrimination, but highlighting ongoing concerns about transparency and fairness in algorithmic advertising.
Example 8: Age-related AI Bias in Healthcare
A notable case of age-related AI bias involved UnitedHealth’s subsidiary NaviHealth, which used an algorithm called nH Predict to determine post-acute care duration.
After 91-year-old Gene Lokken fractured his leg, the algorithm prematurely recommended ending coverage for his nursing home rehabilitation, forcing his family to cover over $12,000 monthly. Critics argued the AI overlooked elderly patients' complex medical needs, disproportionately affecting seniors.
Regulatory agencies, including CMS, emphasized that care decisions must rely on individual assessments, not solely on algorithms.
This incident highlights the necessity for transparency, equitable AI design, and human oversight to prevent bias against older adults in healthcare decisions.
Example 9: Racism + Gender AI Bias
Joy Buolamwini’s Gender Shades project revealed AI biases in the accuracy of commercial facial recognition systems, developed by companies like IBM, Microsoft, and Face. The study found that while error rates for light-skinned males were as low as 0.8%, they soared to 34.7% for dark-skinned females.
Overall, the systems misclassified gender in 1% of white men, but in up to 35% of black women, exposing the heavy bias in datasets used to train these models. Buolamwini’s work not only underscored the ethical risks of deploying AI without representative data but also pressured major tech companies to reevaluate and improve their facial recognition technologies.
Example 10: Twitter’s Racist AI Image Editor
Twitter’s image-cropping algorithm was found to favor white faces over Black faces when automatically generating image previews. In 2020, users began experimenting with side-by-side photos of people of different races, such as Barack Obama and Mitch McConnell, and consistently observed that the algorithm selected the white face for the thumbnail, even when the Black face was more prominent. These experiments went viral, sparking widespread criticism and concern about AI bias in Twitter's machine-learning models.
Twitter eventually acknowledged the issue, stating that its cropping algorithm had been trained to prioritize "salient" image features, but those training choices led to unintended biased outcomes. In response, the company scrapped the automated cropping tool in favor of full-image previews to avoid reinforcing visual discrimination.
Tools and Services to Detect and Eliminate AI Bias
Now that you know AI bias is a real thing, the next question is, is your business suffering from AI bias (unintentionally, of course!), and if yes, how to mitigate it?
Here are some tools that can help detect and eliminate AI biases.
1. Google’s What-If Tool
What it does: A visual, no-code interface to analyze ML model performance.
Details: Google’s What-If Tool (WIT) is an open-source, interactive visualization tool designed to help users explore machine learning models for fairness, performance, and explainability without requiring code.
- Fairness Evaluation: WIT allows users to assess model performance across different demographic groups using various fairness metrics, such as statistical parity and equal opportunity.
- Counterfactual Analysis: Users can modify input features to observe how changes affect model predictions, helping identify potential biases.
- Threshold Adjustment: The tool enables exploration of how changing classification thresholds impacts both accuracy and fairness, facilitating informed decision-making.
- Integration: WIT integrates with TensorBoard and supports models built with TensorFlow, Keras, and other frameworks, making it accessible within existing ML workflows.
Best for: TensorFlow/TFX-based models.
2. Aequitas by the Center for Data Science and Public Policy (University of Chicago)
What it does: Open-source bias and fairness audit tool for classification models.
Details: Aequitas, developed by the Center for Data Science and Public Policy at the University of Chicago, is an open-source toolkit designed to audit machine learning models for bias and fairness. It
- Enables users to assess disparities across demographic groups using metrics such as statistical parity, false positive rate parity, and equal opportunity.
- Supports various interfaces, including a Python library, command-line tool, and web application, making it accessible to data scientists, analysts, and policymakers.
- Generates audit reports showing disparity across groups.
- Helps organizations identify and address discriminatory patterns in predictive models, promoting equitable decision-making in areas like criminal justice, healthcare, and public policy.
Best for: Ideal for government and public sector use.
3. Amazon SageMaker Clarify
What it does: Built-in fairness and explainability tool within AWS SageMaker.
Details: Amazon SageMaker Clarify helps businesses detect and mitigate AI bias by providing tools for fairness analysis and model explainability throughout the machine learning lifecycle. It enables users to:
- Identify Bias: Analyze datasets and models to uncover potential biases related to sensitive attributes like race, gender, or age.
- Understand Predictions: Utilize feature attribution methods, such as SHAP (Shapley Additive Explanations), to interpret how input features influence model predictions.
- Monitor in Production: Continuously track deployed models for bias drift and changes in feature importance over time.
Best for: Teams already using AWS infrastructure.
4. Fiddler AI
What it does: Model performance, explainability, and bias monitoring in production.
Details: Fiddler AI helps businesses mitigate AI bias by offering a comprehensive model monitoring and explainability platform. It enables users to track fairness metrics across different demographic groups, detect performance gaps, and understand model decisions using interpretable explanations.
Fiddler supports continuous monitoring to catch bias drift post-deployment and provides actionable insights to address disparities. It provides Granular insights into why certain groups are misclassified.
Best for: It’s popular in industries like finance, healthcare, and e-commerce.
5. Microsoft Fairlearn
What it does: Helps assess and improve fairness in ML models.
Details: Microsoft Fairlearn is an open-source Python toolkit designed to help developers and data scientists assess and improve the fairness of AI systems. It provides tools to evaluate model performance across different demographic groups and mitigate observed biases.
- Fairness Metrics: Fairlearn offers a suite of metrics to assess fairness in classification and regression tasks. These include demographic parity, equalized odds, and true positive rate parity, allowing users to quantify disparities in model predictions across sensitive attributes like race, gender, or age.
- Mitigation Algorithms: The toolkit includes algorithms such as Exponentiated Gradient and Grid Search to mitigate unfairness by adjusting model predictions or reweighting training data.
- Visualization Dashboard: Fairlearn provides an interactive dashboard to visualize and compare model performance and fairness metrics across different groups, facilitating informed decision-making.
Implementation Steps:
- Installation: Install Fairlearn using pip: Installation Github , Microsoft 1, Microsoft 2
bash
pip install fairlearn
- Data Preparation: Prepare your dataset, ensuring it includes the features, labels, and sensitive attributes you wish to assess for fairness.
- Model Assessment: Use Fairlearn's metrics to evaluate your model's fairness across different groups.
- Bias Mitigation: Apply Fairlearn's mitigation algorithms to address any identified biases.
- Visualization: Utilize the dashboard to visualize the impact of mitigation strategies and make informed decisions.
Fairlearn integrates seamlessly with popular machine learning frameworks like scikit-learn, TensorFlow, and PyTorch, making it a versatile tool for promoting fairness in AI systems.
Best for: Azure ML, scikit-learn.
6. IBM AI Fairness 360 (AIF360)
What it does: Open-source toolkit with over 70 fairness metrics and bias mitigation algorithms.
Details: IBM AI Fairness 360 (AIF360) is an open-source Python toolkit designed to help businesses detect, understand, and mitigate bias in machine learning models. It provides over 70 fairness metrics and 10+ bias mitigation algorithms.
It helps evaluate fairness using metrics like:
- Statistical parity difference
- Disparate impact
- Equal opportunity difference
- Average odds difference
You can run these metrics across different protected attributes like race, gender, or age to find disparities. Tools like fairness dashboards help visualize disparities in model predictions.
Best for: Enterprises using Python-based ML pipelines.
7. Credo AI
What it does: AI governance platform with a focus on risk and compliance.
Overview: Credo AI helps organizations mitigate AI bias by providing a governance platform that monitors and manages AI models for fairness, accountability, and compliance. It evaluates models against internal policies and global regulations, offering tools to assess bias across race, gender, age, and other protected attributes.
The platform integrates with popular ML frameworks and uses standardized scorecards to highlight risks, recommend mitigation strategies, and ensure responsible AI deployment.
Best for: Highly regulated sectors like lending, insurance, and hiring.
Mitigate AI Bias from Customer Support with Crescendo.ai
Crescendo’s next-gen augmented AI is built with high precautions to be free from AI biases. It includes AI-chatbots, AI-powered voice assistance, automated email ticket support, knowledgebase management, AI-based CX insights, compliance and QA handling, and much more. Book a demo to explore more.